Data science is an ever-evolving realm, as businesses across all sectors look for different ways to use important information to stay ahead of competitors or develop new trends. One of the ways in which this is being accomplished is through what’s known as an analysis of variance, or ANOVA. This is a range of scenarios used to determine if there is a difference between the means of different groups based on independent variables and dependent variables in different settings. Here are just some of the ways that ANOVA tests have found their way into the business world.
1. Medical Research
You may still be asking yourself, what is an ANOVA? It’s a statistical formula that uncovers a ratio, known as an “F statistic,” showing the difference between the within-group variance and the between-group variance. This ultimately produces a figure that allows a conclusion that the null hypothesis is supported or rejected. If there’s a significant difference, the F-ratio will be larger and draw attention to a statistical significance.
For example, ANOVA tests have become quite commonplace in medical research to uncover single factors in the innovation of life-saving medication or treatments. ANOVA allows scientists and researchers to explore relationships between different types of medicine and blood levels across a test group of patients. This statistical method can reveal where the distribution of a particular drug is beneficial, such as to a particular age group. It can use these descriptive statistics to take on different factors that draw faster and better hypotheses on the development process.
2. Seasonal Trends
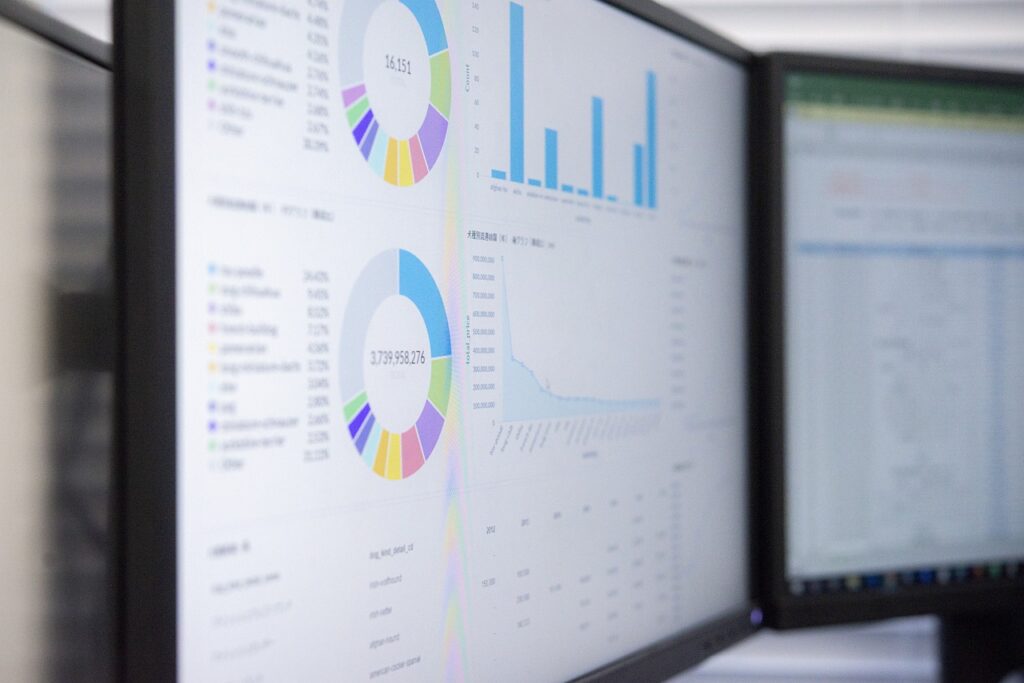
In different lines of industry, the time of year can demonstrate what drives annual sales or product innovation. Retailers, for example, turn to ANOVA tests to spot what is driving the margins based on random factors, such as seasonality, age group, or pricing. ANOVA allows these retailers to avoid replication of data that throws off their basic analytics, while better understanding the critical values of certain products and services based on trends. Having this real-time data analysis and the ability to comparison test makes for better business decisions.
ANOVA takes into account the overall mean based on a given time frame or other random factors to analyze what’s working, what isn’t, what can be changed, and what should be. This can be accomplished through two-way ANOVA, also known as full factorial ANOVA, which takes into account multiple independent variables for monitoring. ANOVA helps to find out if the difference in the mean values is statistically significant. This allows statisticians to spot any deviations amongst retailers, as well as other outliers in these industries.
3. Manufacturing
One of the biggest challenges in machine learning and data science is the selection of the most reliable and useful features to train an ML-based model. ANOVA helps in selecting the best features, minimizing the number of input variables to reduce the complexity of an ANOVA model. ANOVA helps to determine if an independent variable is influencing a target variable, affording an understanding of different sources of variation with the mean of each group based on independent samples.
This is a tremendous asset for manufacturers and those who rely on these processes to benefit their supply chain. Through one-way ANOVA tests or single-factor ANOVA, businesses can uncover a significant F-statistic that is slowing down development, in turn slowing down sales. The ability to spot where on average there are stalls in workflow and downtime provides manufacturing companies with a baseline to make judgment calls to benefit an organization internally. This in turn contributes to research design on certain products, and any additional tests that provide a more streamlined, linear model to build for the future.